基于强迫症核心家系全基因组的致病突变研究:
在这个项目里,我们对一群患有早期强迫症的患者和他们无强迫症症状的父母 (样本由医学院附属精神卫生中心采集)进行核心家系Trio全基因组测序。 对所捕获的突变大数据组进行有效生信统计,对比分析检测新生突变,再结合多组学多维度数据, 利用机器学习方法去构建一个预测强迫症致病因子的计算模型。 我们将通过与患者的临床表型关联,对预测出的高风险因子分析其和脑功能通路的关联以及它们的致病原理。 我们希望通过本项目的研究能识别出一组影响强迫症患者脑功能的风险基因和致病性突变.
WGS study on the genetics of Obsessive-Compulsive Disorder (OCD):
In this project, we are performing the whole-genome sequencing (WGS) of a cohort of patients diagnosed with early-onset Obsessive-Compulsive Disorder (OCD) together with their unaffected parents (collected by Shanghai Mental Health Center), where we hope to identify de novo mutations and risk genes that involve in the etiology of the disease. We hope that research in this area will eventually help to identify the pathogenic mutations and disease pathways, and to further refine the categorization of disease pathology, so that therapies can be more targeted and effective.
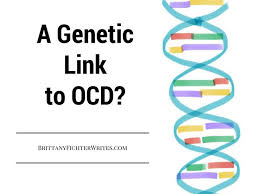
综合基因网络对精神分裂症(SCZ)和双向情感障碍(BP)的共病研究:
精神分裂症主要为精神障碍;躁郁症主要为的情绪障碍,但也可能涉及精神病。但是,由于两者有着一些类似的临床症状,区分两者有时会很困难。 而事实上,确实存在中度分裂情感性精神障碍,所以此研究重在研究两者的共病机制。而常见的多效机制有可能就是SCZ和BP共病的基础。 我们将根据构建共病功能通路和基因网络来确定SCZ和BP之间的“遗传重叠”的程度。 可帮助深入了解这两种在基因上相互相关,临床表型有重叠,但仍然是不同的神经发育障碍的内在病理机制。我们将使用生物信息学的方法来 构建综合遗传网络,包括新生突变数据,高风险CNV,microRNA数据,动物模型和基因表达图谱。我们旨在预测与SCZ 和BP遗传相关的神经发育障碍的遗传通路和遗传网络,并辨别常见的多效性遗传可作为药物靶标的风险变体。
Integrated genetic network study of comorbidity between Schizophrenia(SCZ) and Bipolar(BP)
Common pleiotropic mechanisms may underlie shared repetitive symptoms across SCZ and BP. In this project, we will determine the extent of "genetic overlap" in terms of shared underlying gene pathways and gene networks between SCZ and BP, to provide further insights into how aberrant processes underlie these genetically related, clinically overlapping but still distinct neurodevelopmental disorders. We will use a combination of bioinformatics and literature approaches to construct integrated genetic networks in-corroborating genetic evidence including de novo mutation data and recurrent high risk ‘genome-wide’ CNVs, microRNA data, animal models and gene expression studies. We aim to Detect and integrate common genetic pathways and genetic networks for neurodevelopmental disorders that are genetically related to SCZ and/or BP, and to identify common pleiotropic genetic risk variants as possible druggable targets.
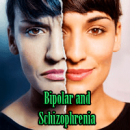
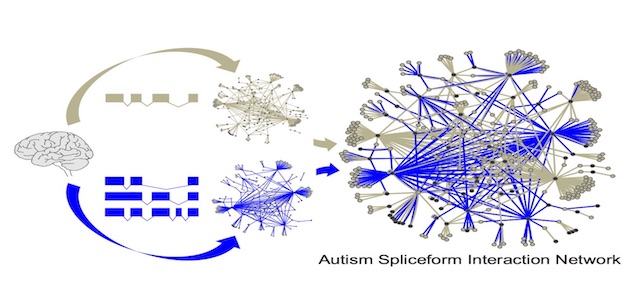
建立中国人群SCZ、BP分子-影像生物标志物图谱数据库,搭建多组学、脑影像精准分析云平台:
在这个项目里,我们将搭建一个中央化的中国人群SCZ、BP分子-影像生物标志物大数据存储和备份平台,以及大数据分析平台。 实现对海量数据的统一存储、分析与共享。这个云平台将在科研和临床上实现以下两大目标:在科研上提供中国人群SCZ和BP图谱数据库的共享。 科研用户可以用简单的网络方式登录并访问我们课题整合或新发现的SCZ、BP的影像学、生物标志物等图谱数据库,并提供原始数据的下载; 在临床上,根据病人多模态信息提供一个精准诊疗方案。临床终端用户可以用简单的网络方式登录并上传我们所认可的病人的多模态图谱数据, 经过不依赖终端用户的远程云端数据处理,返回一个精准诊疗方案。
Build a -OMIC knowledge base of SCZ and BP Chinese cohort
In this National Key Research and Development Program of China supported project, we are building a cloud knowledge-base of -omic data and brain MRI images of SCZ and BP patient cohorts. It will be a centralized big data analysis platform and big data storage and backup platform as the unified storage, analysis and sharing of massive data.
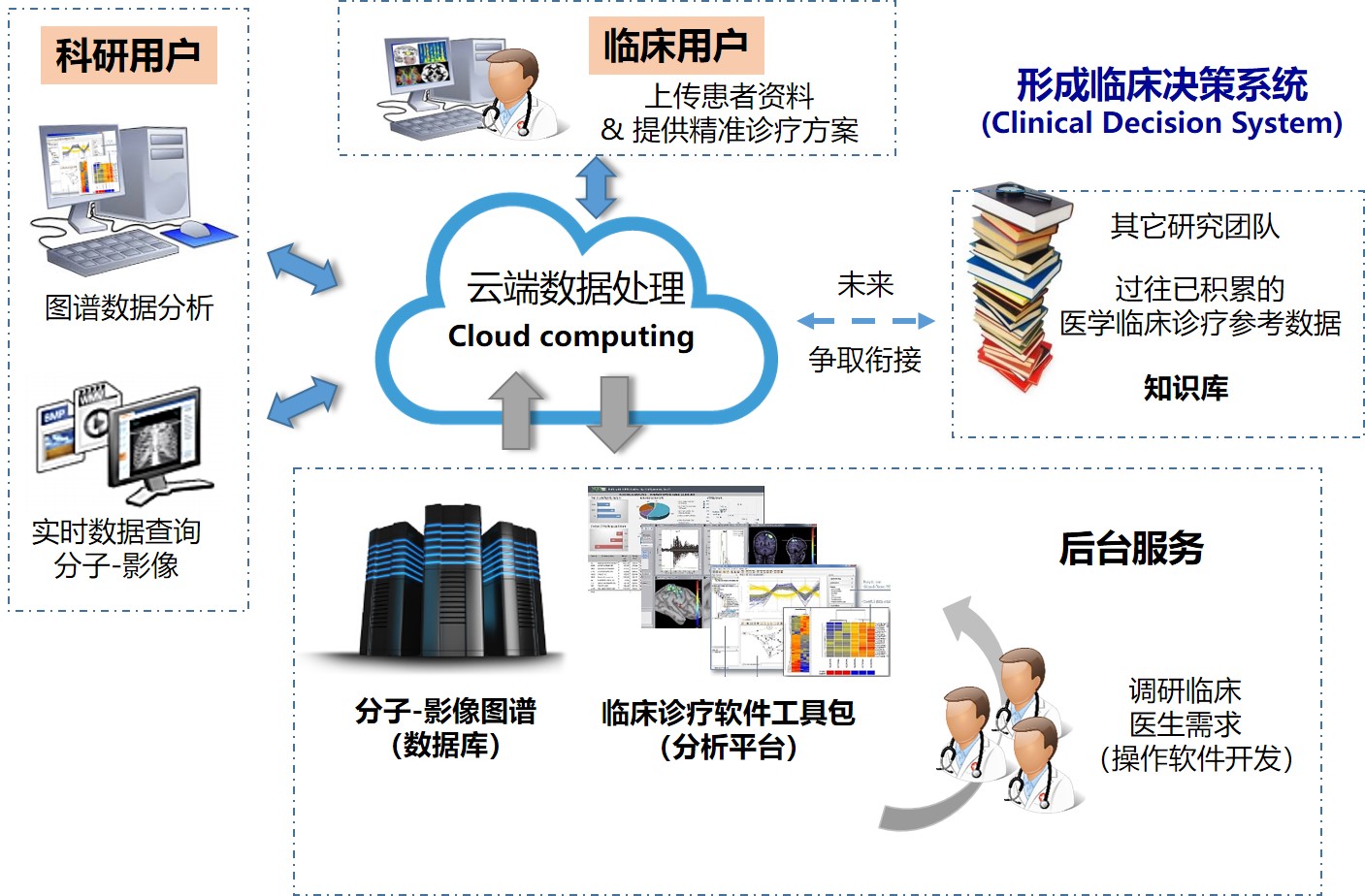
条件性敲除自闭症风险基因小鼠模型的研究:
虽然在识别自闭症和其他神经发育障碍的遗传原因,以及这些疾病潜在分子机制认识方面,目前已有了很大的进展。 然而,我们在被遗传突变所破坏的神经分子通路,以及能否有个性化的精准治疗上,认识仍然相对有限。 因此携带着人类基因突变的小鼠模型是作为能提高我们对这些神经分子通路认知的重要工具。在这些项目中,我们将通过构建条件性敲自闭症风险基因的小鼠模型 来研究疾病病因并试图了解其脑功能分子通路径。我们将利用这些小鼠模型来研究基因突变对神经细胞,不同脑区的转录和翻译水平的影响。 我们的目标是揭开与疾病表型相关的细胞和分子机制。
Autism genes conditional knockout mouse model studies
The progress in identifying genetic causes of autism and other neurodevelopmental disorders has opened new possibilities for improving our understanding of the molecular mechanisms underlying these diseases. However, the knowledge of the molecular pathways that are disrupted by genetic mutations, and of those that could be targeted therapeutically, remains quite limited. Mouse models that carry human genetic mutations serve as important tools for improving the knowledge about these pathways. In these projects, we are making conditional autism gene knockout mouse models to study the disease etiology and try to understand the underlying molecular pathways.We are investigating the impact of these mutations at the cellular, transcriptional and translational levels using developing mouse brain. Our goal is to unravel detailed cellular and molecular mechanisms responsible for the disease phenotypes.
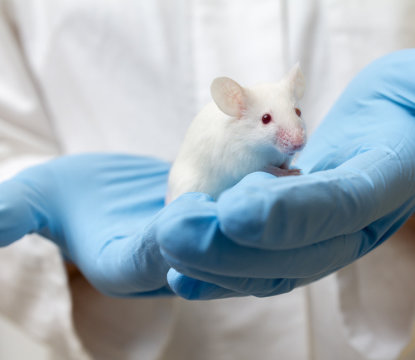
其它科研项目还包括基因组学方面的数据挖掘(机器学习)的转化医学研究,我们不间断的和校内外合作者开拓新的合作项目。
Other areas of interest include machine learning in the context of genomics and translational genetics. We are constantly forming on and off-campus collaborations to further these and other areas of interest.